Algorithms and applications for Gaussian graphical models under the multivariate totally positive constraint of order 2
Author(s)
Roy, Uma,M. Eng.Massachusetts Institute of Technology.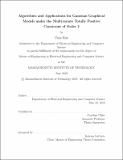
Download1220877228-MIT.pdf (961.8Kb)
Other Contributors
Massachusetts Institute of Technology. Department of Electrical Engineering and Computer Science.
Advisor
Caroline Uhler.
Terms of use
Metadata
Show full item recordAbstract
We consider the problem of estimating an undirected Gaussian graphical model when the underlying distribution is multivariate totally positive of order 2 (MTP₂), a strong form of positive dependence. A large body of methods have been proposed for learning undirected graphical models without the MTP₂ constraint. A major limitation of these methods is that their consistency guarantees in the high-dimensional setting usually require a particular choice of a tuning parameter, which is unknown a priori in real world applications. We show that an undirected graphical model under MTP₂ can be learned consistently without any tuning parameters. We evaluate this new estimator on synthetic and real-world financial data sets, showing that it out-performs other methods in the literature with tuning parameters. We further explore applications of estimators in the MTP₂ setting to covariance estimation for finance. In particular, the very well-explored optimal Markowitz portfolio allocation problem requires a precise estimate of the covariance matrix of returns. By exploiting the fact that the returns of assets are typically positively dependent, we propose a new estimator based on MTP₂ estimation and show that this estimator outperforms (in terms of out-of-sample risk) baseline methods including shrinkage techniques and explicitly providing market factors on stock-market data spanning over thirty years.
Description
This electronic version was submitted by the student author. The certified thesis is available in the Institute Archives and Special Collections. Thesis: M. Eng., Massachusetts Institute of Technology, Department of Electrical Engineering and Computer Science, June, 2019 Cataloged from student-submitted PDF of thesis. Includes bibliographical references (pages 67-72).
Date issued
2019Department
Massachusetts Institute of Technology. Department of Electrical Engineering and Computer SciencePublisher
Massachusetts Institute of Technology
Keywords
Electrical Engineering and Computer Science.