Deep learning methods for the design and understanding of solid materials
Author(s)
Xie, Tian,Ph.D.Massachusetts Institute of Technology.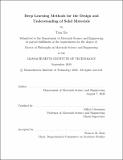
Download1227037031-MIT.pdf (18.91Mb)
Other Contributors
Massachusetts Institute of Technology. Department of Materials Science and Engineering.
Advisor
Jeffrey Grossman.
Terms of use
Metadata
Show full item recordAbstract
The trend of open material data and automation in the past decade offers a unique opportunity for data-driven design of novel materials for various applications as well as fundamental scientific understanding, but it also poses a challenge for conventional machine learning approaches based on structure features. In this thesis, I develop a class of deep learning methods that solve various types of learning problems for solid materials, and demonstrate its application to both accelerate material design and understand scientific knowledge. First, I present a neural network architecture to learn the representations of an arbitrary solid material, which encodes several fundamental symmetries for solid materials as inductive biases. Then, I extend the approach to explore four different learning problems: 1) supervised learning to predict material properties from structures; 2) visualization to understand structure-property relations; 3) unsupervised learning to understand atomic scale dynamics from time series trajectories; 4) active learning to explore an unknown material space. In each learning problem, I demonstrate the performance of the approach compared with previous approaches, and apply it to solve several realistic materials design problems and extract scientific insights from data.
Description
Thesis: Ph. D., Massachusetts Institute of Technology, Department of Materials Science and Engineering, 2020 Cataloged from student-submitted PDF of thesis. Includes bibliographical references (pages 127-145).
Date issued
2020Department
Massachusetts Institute of Technology. Department of Materials Science and EngineeringPublisher
Massachusetts Institute of Technology
Keywords
Materials Science and Engineering.