Algorithmic intervention to mitigate inventory and ordering amplification in multi-echelon supply chains
Author(s)
Paine, James Edward.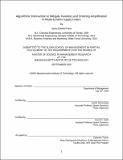
Download1227106413-MIT.pdf (1003.Kb)
Other Contributors
Sloan School of Management.
Advisor
Hazhir Rahmandad and David R. Keith.
Terms of use
Metadata
Show full item recordAbstract
The 'bullwhip effect' is a classic, yet persisting, problem with reverberating consequences in inventory management and refers to how forecast errors and safety stock builds yield increasing amplitudes in both orders and on-hand inventory positions the further one moves away from a source of order variability. The bullwhip effect is responsible for both excessive strain on real world inventory management systems, stock outs, and unnecessary capital reservation though safety stock building. In this paper, the author develops algorithmic approaches to mitigating bullwhip using simulation modeling, including cost minimization and amplification minimization, and then interprets the results in the context of existing models of human heuristics in ordering decisions. The algorithmic approaches are optimized as one member within a model of a human decision makers operating within a multi-echelon supply chain with imperfect information sharing and information delays. Within the optimization, human decision biases such as supply line under-weighting are compensated for by the developed methods via the control of the flow of information and simulated physical goods both up and downstream. In all methods developed, inventory and ordering oscillations are minimized in the simulated environment. The overall goal of this project is to develop useful, implementable, and (to the degree possible) understandable algorithms capable of mitigating bullwhip generated by real humans when placed into an actively evolving inventory management crisis in-progress. To this end, the parameters that emerge in the developed algorithm are mapped to previously observed modes of behavior that mitigate the effects of bullwhip. The resulting algorithms act in a manner analogous to those exhibiting high levels of trust within the supply chain, coupled with a cautious approach to information signals outside of the supply chain. Desired stock levels of the resulting algorithms approach those found in optimal base-stock replenishment policies. Finally, it is observed that the algorithm does not fall prey to supply line under-weighting and can act to offset the ordering decisions that typically result in bullwhip in a simulated model of a multi-echelon supply chain.
Description
Thesis: S.M in Management Research, Massachusetts Institute of Technology, Sloan School of Management, September, 2020 Cataloged from student-submitted PDF version of thesis. Includes bibliographical references (pages 37-39).
Date issued
2020Department
Sloan School of ManagementPublisher
Massachusetts Institute of Technology
Keywords
Sloan School of Management.