Learned functions for perceptually informed robot navigation
Author(s)
Stadler, Martina Katherine.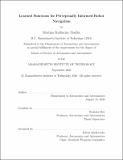
Download1227504264-MIT.pdf (4.505Mb)
Other Contributors
Massachusetts Institute of Technology. Department of Aeronautics and Astronautics.
Advisor
Nicholas Roy.
Terms of use
Metadata
Show full item recordAbstract
While existing robotic systems predominantly rely on geometric information to inform robot navigation, non-geometric information, such as object-level maps and overhead imagery, provide rich navigation cues that can be used to inform intelligent navigation behaviors. However, it is not obvious how non-geometric navigation cues should be incorporated into existing robot motion planning pipelines. This thesis presents two novel methods that use learning to incorporate nongeometric information into classical planning techniques for robot navigation. First, we present Learned Sampling Distributions, a novel method for learning a sampling distribution based on local hybrid geometric and object-level maps to inform a sampling-based motion planner for navigation in unknown environments. Our approach uses expert demonstrations to learn a probability distribution that places high probability in regions of the environment that are likely to be on optimal paths to the goal, like hallways and doorways in an office environment, and results in up to a 2.7x increase in the probability of finding a plan for a resource-constrained agent when compared to a baseline planner. Second, we present Perceptually Informed Abstractions, a novel method for hierarchical planning at long length scales that learns properties of abstract actions for use in a risk-aware hierarchical discrete planner, conditioned on low-resolution overhead images. We also present a preliminary analysis of the approach in a simulated toy environment.
Description
Thesis: S.M., Massachusetts Institute of Technology, Department of Aeronautics and Astronautics, September, 2020 Cataloged from student-submitted PDF of thesis. Includes bibliographical references (pages 101-109).
Date issued
2020Department
Massachusetts Institute of Technology. Department of Aeronautics and AstronauticsPublisher
Massachusetts Institute of Technology
Keywords
Aeronautics and Astronautics.