Reorienting machine learning education towards tinkerers and ML-engaged citizens
Author(s)
Lao, Natalie.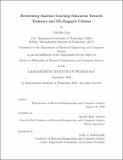
Download1227520621-MIT.pdf (36.11Mb)
Other Contributors
Massachusetts Institute of Technology. Department of Electrical Engineering and Computer Science.
Advisor
Harold (Hal) Abelson.
Terms of use
Metadata
Show full item recordAbstract
Artificial intelligence (AI) and machine learning (ML) technologies are appearing in everyday contexts, from recommendation systems for shopping websites to self -riving cars. As researchers and engineers develop and apply these technologies to make consequential decisions in everyone's lives, it is crucial that the public understands the AI-powered world, can discuss AI policies, and become empowered to engage in shaping these technologies' futures. Historically, ML application development has been accessible only to those with strong computational backgrounds working or conducting research in highly technical fields. As ML models become more powerful and computing hardware becomes faster and cheaper, it is now technologically possible for anyone with a laptop to learn about and tinker with ML. This includes audiences without programming or advanced mathematics knowledge, who can use public ML tools to construct both useful technical artifacts and meaningful socio-ethical artifacts around ML. My research investigates the following question: How do we reorient ML engineering education to transform "ML consumers" to be "ML contributors"? In order to illuminate this problem, I have created a Machine Learning Education Framework. In this dissertation, I present the framework and three applications of it: (1) a course based on the framework that aims to develop ML self-efficacy in general college-level audiences; (2) a curriculum rubric based on the ML Education Framework and analyses of 6 ML/AI courses using the rubric; and (3) two public ML tools for high school and above ML tinkerers that empower them to create personal ML applications through image and audio classification. The ML Education Framework is organized into three interconnected categories: Knowledge (General ML Knowledge, Knowledge of ML Methods, Bias in ML Systems, Societal Implications of ML), Skills (ML Problem Scoping, ML Project Planning, Creating ML Artifacts, Analysis of ML Design Intentions & Results, ML Advocacy, Independent Out-of-Class Learning), and Attitudes (Interest, Identity & Community, Self-Efficacy, Persistence). The three top level categories of the framework were chosen to encompass the holistic process of teaching people ML and ensuring continued participation in the field. "Knowledge" about ML will be necessary for individuals to thrive in today's ML-driven world and builds foundations for further engagement with the technology. ML "Skills" define specific actions and activities that students need to do and practice in order to become ML contributors. The category "Attitudes" reflects long-term societal goals for educational initiatives in ML, and addresses the perspectives that students should develop through an ML course. This framework can be used in creating new ML curricula, in analyzing the results of ML interventions, and in situating support tools within ML education for students of a variety of backgrounds and levels. Today a large portion of the world solely resides in the "ML consumer" population. Regulation of ML technologies is nascent. As policymakers think about reasonable legal structures, the public should be able to actively participate in these discussions. It is imperative that we empower these "ML consumers" to become "ML tinkerers" and "ML-engaged citizens".
Description
Thesis: Ph. D., Massachusetts Institute of Technology, Department of Electrical Engineering and Computer Science, September, 2020 Cataloged from student-submitted PDF of thesis. Includes bibliographical references (pages 213-223).
Date issued
2020Department
Massachusetts Institute of Technology. Department of Electrical Engineering and Computer SciencePublisher
Massachusetts Institute of Technology
Keywords
Electrical Engineering and Computer Science.