Geometric matrix completion with graph attention networks
Author(s)
Ruiz, Rodrigo I.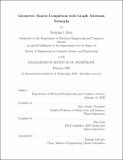
Download1237564537-MIT.pdf (1.630Mb)
Other Contributors
Massachusetts Institute of Technology. Department of Electrical Engineering and Computer Science.
Advisor
Alex 'Sandy' Pentland and Yan Leng.
Terms of use
Metadata
Show full item recordAbstract
Many real-world data cover multiple aspects of consumer behaviors and business characteristics, creating opportunities for marketing companies to better capture the demand and preferences of customers with complementary information. However, to effectively combine data with multi-modal nature and complex structure is challenging. In this thesis, we propose a novel geometric deep learning framework for building effective recommender systems by predicting customers' preferences on businesses they have not yet rated. The proposed framework is capable of handling heterogeneous and auxiliary information on businesses and customers, and at the same time enforcing that only information relevant to the prediction task will be utilized. We compare the proposed framework with several baseline models in a prediction task using the Yelp open data set, where the improved performance of our method highlights the advantage of incorporating spatial, temporal, network, and other types of data in a principled manner. The proposed framework can be further applied to help make more informed marketing and managerial decisions in a variety of domains where the fusion of heterogeneous and structured information could be beneficial.
Description
Thesis: M. Eng., Massachusetts Institute of Technology, Department of Electrical Engineering and Computer Science, February, 2020 Cataloged from student-submitted PDF of thesis. Includes bibliographical references (pages 47-50).
Date issued
2020Department
Massachusetts Institute of Technology. Department of Electrical Engineering and Computer SciencePublisher
Massachusetts Institute of Technology
Keywords
Electrical Engineering and Computer Science.