Efficient homomorphically encrypted privacy-preserving automated biometric classification
Author(s)
Stein, David Benjamin.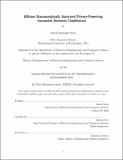
Download1249684928-MIT.pdf (2.305Mb)
Other Contributors
Massachusetts Institute of Technology. Department of Electrical Engineering and Computer Science.
Advisor
Daniela Rus.
Terms of use
Metadata
Show full item recordAbstract
This thesis investigates whether biometric recognition can be performed on encrypted data without decrypting the data. Borrowing the concept from machine learning, we develop approaches that cache as much computation as possible to a pre-computation step, allowing for efficient, homomorphically encrypted biometric recognition. We demonstrate two algorithms: an improved version of the k-ishNN algorithm originally designed by Shaul et. al. in [1] and a homomorphically encrypted implementation of a SVM classifier. We provide experimental demonstrations of the accuracy and practical efficiency of both of these algorithms.
Description
Thesis: M. Eng., Massachusetts Institute of Technology, Department of Electrical Engineering and Computer Science, September, 2020 Cataloged from the official PDF of thesis. Includes bibliographical references (pages 87-96).
Date issued
2020Department
Massachusetts Institute of Technology. Department of Electrical Engineering and Computer SciencePublisher
Massachusetts Institute of Technology
Keywords
Electrical Engineering and Computer Science.