The acceptability delta criterion : memorization is not enough
Author(s)
Vázquez Martínez, Héctor Javier.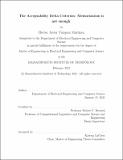
Download1251800552-MIT.pdf (2.290Mb)
Other Contributors
Massachusetts Institute of Technology. Department of Electrical Engineering and Computer Science.
Advisor
Robert C. Berwick.
Terms of use
Metadata
Show full item recordAbstract
In order to effectively assess Knowledge of Language (KoL) for any statistically-based Language Model (LM), one must develop a test that is first comprehensive in its coverage of linguistic phenomena; second backed by statistically-vetted human judgement data; and third, tests LMs' ability to track human gradient sentence acceptability judgements. Presently, most studies of KoL on LMs have focused on at most two of these three requirements at a time. This thesis takes steps toward a test of KoL that meets all three requirements by proposing the LI-Adger dataset: a comprehensive collection of 519 sentence types spanning the field of generative grammar, accompanied by attested and replicable human acceptability judgements for each of the 4177 sentences in the dataset, and complemented by the Acceptability Delta Criterion (ADC), an evaluation metric that enforces the gradience of acceptability by testing whether LMs can track the human data. To validate this proposal, this thesis conducts a series of case studies with Bidirectional Encoder Representations from Transformers (Devlin et al. 2018). It first confirms the loss of statistical power caused by treating sentence acceptability as a categorical metric by benchmarking three BERT models fine-tuned using the Corpus of Linguistic Acceptability (CoLA; Warstadt & Bowman, 2019) on the comprehensive LI-Adger dataset. We find that although the BERT models achieve approximately 94% correct classification of the minimal pairs in the dataset, a trigram model trained using the British National Corpus by Sprouse et al. 2018, is able to perform similarly well (75%). Adopting the ADC immediately reveals that neither model is able to track the gradience of acceptability across minimal pairs: both BERT and the trigram model only score approximately 30% of the minimal pairs correctly. Additionally, we demonstrate how the ADC rewards gradience by benchmarking the default BERT model using pseudo log-likelihood (PLL) scores, which raises its score to 38% correct prediction of all minimal pairs. This thesis thus identifies the need for an evaluation metric that tests KoL via gradient acceptability over the course of two case studies with BERT and proposes the ADC in response. We verify the effectiveness of the ADC using the LI-Adger dataset, a representative collection of 4177 sentences forming 2394 unique minimal pairs each backed by replicable and statistically powerful human judgement data. Taken together, this thesis proposes and provides the three necessary requirements for the comprehensive linguistic analysis and test of the Human KoL exhibited LMs that is currently missing in the field of Computational Linguistics.
Description
Thesis: M. Eng., Massachusetts Institute of Technology, Department of Electrical Engineering and Computer Science, February, 2021 Cataloged from the official PDF of thesis. Includes bibliographical references (pages 71-73).
Date issued
2021Department
Massachusetts Institute of Technology. Department of Electrical Engineering and Computer SciencePublisher
Massachusetts Institute of Technology
Keywords
Electrical Engineering and Computer Science.