Computational tools towards automating the scientific method
Author(s)
Spanbauer, Span.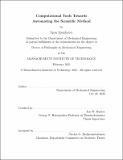
Download1252628873-MIT.pdf (7.770Mb)
Other Contributors
Massachusetts Institute of Technology. Department of Mechanical Engineering.
Advisor
Ian W. Hunter.
Terms of use
Metadata
Show full item recordAbstract
We present a collection of novel computational tools designed to contribute to the goal of large-scale scientific automation. Deep Involutive Neural MCMC and other inference compilation techniques present a promising path to accelerating inference in probabilistic programs. Neural Group Actions provide foundational methods for learning symmetric transformations useful for the development of statistical models and probabilistic algorithms. Coarse-Grained Nonlinear System Identification provides an exceptional new model class for nonlinear dynamic systems, enabling accurate model identification with minimal experimental data. Optimization plus Stochastic Interchange is a flexible new way to generate experimental stimuli, leading to optimally informative measurements during system identification. Extended Koopman Models advance a new method for the optimal control of nonlinear systems. When coupled with high-throughput laboratory automation, these and other computational tools made possible by recent developments in artificial intelligence promise to revolutionize the way we do science and engineering.
Description
Thesis: Ph. D., Massachusetts Institute of Technology, Department of Mechanical Engineering, February, 2021 Cataloged from the official PDF of thesis. Includes bibliographical references (pages 123-134).
Date issued
2021Department
Massachusetts Institute of Technology. Department of Mechanical EngineeringPublisher
Massachusetts Institute of Technology
Keywords
Mechanical Engineering.