Learning to trust in forecast information sharing
Author(s)
Zhang, Pengbo, S.M. Massachusetts Institute of Technology.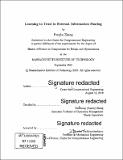
Download1281184133-MIT.pdf (5.678Mb)
Other Contributors
Massachusetts Institute of Technology. Computation for Design and Optimization Program.
Terms of use
Metadata
Show full item recordAbstract
This thesis follows and extends the discussion of Özer et al. (2011) on trust in forecast information sharing. We propose a method for belief learning and for updating. The effects of production cost (which indicate the risk) and market uncertainty (which indicates the accuracy of the private information) are analyzed quantitatively. Since complicated Nash equilibria from traditional game theory analysis often fail in real-life scenarios, we formulate simpler assumptions so that the strategies of both sides are not complicated. We compare the similarities and differences between the structure of our model and the structure of other behavioral models related to bounded rationality or cheap talk. We characterize how the supply chain environment changes trust and decisions. We find out that initial beliefs do not matter because they will be quickly adjusted by the market: the limiting behavior, as t --> [infinity], depends only on the retailers' trustworthiness and supply chain environment. Since the retailer's trustworthiness and belief is un-observable, we perform latent profile analysis to fit the model on the experiment conducted by Özer et al. (2011), and test the end game effect and out-of-sample fit.
Description
Thesis: S.M., Massachusetts Institute of Technology, Computation for Design and Optimization Program, September, 2019 Manuscript. Includes bibliographical references (pages 93-94).
Date issued
2019Department
Massachusetts Institute of Technology. Computation for Design and Optimization ProgramPublisher
Massachusetts Institute of Technology
Keywords
Computation for Design and Optimization Program.