Enhancing methods for modeling and estimation of complex socio-technical systems
Author(s)
Li, Tianyi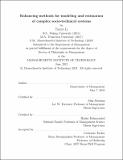
DownloadThesis PDF (5.917Mb)
Advisor
Sterman, John
Rahmandad, Hazhir
Terms of use
Metadata
Show full item recordAbstract
This thesis consists of three studies designed to improve the rigor of simulation models of complex dynamic systems in social sciences and to enhance the use of quantitative data in the estimation of model structure and parameters. The data available to social scientists developing models of complex systems are often severely limited and suffer from substantial measurement error. Acquiring additional data can be difficult and expensive. Existing methods to estimate model structure and parameters, and to test these models, are often inappropriate. Each essay in this thesis addresses an important dimension of these issues.
Chapter 1: We study parameter estimation methods in the context of epidemic models. We compare standard least-squares estimation with a panel of alternative estimation schemes in which we test various likelihood functions as well as the use of Kalman filtering. We explore the performance of these methods under different assumptions about data availability and quality, including missing data on important variables and measurement error. While all methods perform comparably in terms of bias in estimated parameters, they vary significantly in the quality of the confidence intervals they yield. Naive least-squares estimation performs poorly, while a negative binomial likelihood or the application of Kalman filtering yields more reliable results. The results should apply not only to epidemics, but to models of social contagion, innovation adoption and diffusion, and potentially other domains.
Chapter 2: When sufficient data for model specification and estimation are unavailable, how should modelers optimally determine which data should be acquired? Specifically, for a given model and set of variables to collect data on, which next k model variables provide the greatest utility for model calibration? We connect this problem with the sensor placement problem in engineering systems, which leads to a combinatorial optimization. We first translate two established solution approaches from engineering systems to social science simulation models. Then, based on the idea of Data Availability Partition and drawing on insights from existing solutions, we propose a new objective function for the optimization. Analytical results for the optimal placement solution under the new objective function are derived for binary and multi-ary trees. For a general tree structure with n nodes, the optimal placement algorithm is devised, with complexity growing at an upper bound of O(nlog2(n)). For arbitrary model structures with feedback loops, approximate solution schemes are developed. Comparison against existing approaches shows notable advantages of the newly proposed method. These findings provide modelers across domains with an objective method and a useful toolkit to prioritize data acquisition.
Chapter 3: Because System Dynamics (SD) strives to create realistic, operationally grounded, endogenous explanations of broad-boundary issues, it often needs large complex models that are difficult to understand and leverage at the aggregate level. Recent efforts have formalized the analysis on the structural determinants of the system’s transient behavior. In this study, we complement these efforts by focusing on model elements (i.e., control inputs) that are ultimately responsible for managing the levels of system’s state variables. We borrow from structural control theory and develop a set of analysis to formally identify the control inputs in a model and assess their capacity to control system states. This post-modeling workflow is summarized as the structural control analysis (SCA) of SD models. The results of these algorithms provide insights into the system controllability and policy design. We illustrate these benefits through several examples and outline potential areas of future research.
Date issued
2021-06Department
Sloan School of ManagementPublisher
Massachusetts Institute of Technology