Meta-Learning and Self-Supervised Pretraining for Few-shot Image Translation
Author(s)
Rugina, Ileana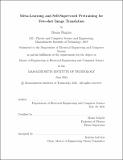
DownloadThesis PDF (2.762Mb)
Advisor
Soljačić, Marin
Terms of use
Metadata
Show full item recordAbstract
Recent advances in machine learning (ML) and deep learning in particular, enabled by hardware advances and big data, have provided impressive results across a wide range of computational problems such as computer vision, natural language, or reinforcement learning. Many of these improvements are however constrained to problems with large-scale curated data-sets which require a lot of human labor to gather. Additionally, these models tend to generalize poorly under both slight distributional shifts and low-data regimes. In recent years, emerging fields such as meta-learning or self-supervised learning have been closing the gap between proof-of-concept results and real-life applications of ML.
We follow this line of work and contribute a novel few-shot multi-task image to image translation problem. We then present several benchmarks for this problem using ideas from both meta-learning and contrastive-learning and improve upon baselines trained using simple supervised learning. Additionally, we contribute to another area of growing interest—applying deep learning to physical problems—and focus our efforts on modeling weather phenomena.
We define an image translation problem between different radar and satellite sensor modalities and leverage spatial and temporal locality to pose it as a multi-task problem. We improve upon naive solutions that ignore this hierarchical dataset structure and demonstrate the effectiveness of meta-learning methods to solving real-world problems. We make our code available here.
Date issued
2021-06Department
Massachusetts Institute of Technology. Department of Electrical Engineering and Computer SciencePublisher
Massachusetts Institute of Technology