Outlier-Robust Multi-View Triangulation Using Graduated Non-Convexity for Space Vehicle Navigation
Author(s)
Mitchell, Adriana Macieira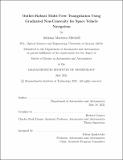
DownloadThesis PDF (3.635Mb)
Advisor
Linares, Richard
Terms of use
Metadata
Show full item recordAbstract
Triangulation is used in terrain relative navigation (TRN) to identify the position of terrain features with respect to the spacecraft and to ultimately estimate the spacecraft’s pose during planetary landing and navigation. This thesis seeks to improve current multi-view triangulation methods to optimally perform with an outlier-dominant (>50% outlier) dataset. Outlier-dominant situations may occur in cases of extreme environmental changes (poor lighting conditions, obscured vision), unreliable sensors, and in the case of TRN, incorrect feature matching. We show that current multi-view triangulation solvers fail at >=10% outlying measurements. We apply Yang et al.’s (2020) Graduated Non-Convexity (GNC) algorithm to three chosen multi-view triangulation solvers and improve the best performing solver’s robustness to 50% outliers which outperforms the current state-of-the-art outlier removal method RANSAC. We apply the robust multi-view triangulation solver to a simulated lunar landing trajectory and reported the variance of the returned 3D error to verify the accuracy of the estimate. This new technique of making current multi-view triangulation solver robust to outliers using GNC provides exciting performance and displays promise for future implementation on a terrain relative navigation system. This combination has not been shown before in previous work, but this thesis enables the conclusion that outlier-robust methods can be applied to TRN.
Date issued
2021-06Department
Massachusetts Institute of Technology. Department of Aeronautics and AstronauticsPublisher
Massachusetts Institute of Technology