Predicting Individual Components of the SOFA Score using Multi-Task Learning
Author(s)
Yang, Alexander Y.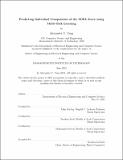
DownloadThesis PDF (875.8Kb)
Advisor
Guttag, John
Syed, Zeeshan
Saeed, Mohammed
Terms of use
Metadata
Show full item recordAbstract
The Sequential Organ Failure Assessment (SOFA) score is a scoring system useful for predicting clinical outcomes in the intensive care unit, such as organ failure and mortality. The availability of increasingly detailed electronic clinical data has allowed for the creation of more powerful models to better predict and improve patient outcomes. However, there is little work in predicting the underlying physiological measurements that define the SOFA score. In this paper, we consider predicting changes to the individual components of the SOFA score. We use multi-task learning frameworks to predict future values for the SOFA score components, with the goal of sharing information across the different tasks to improve overall predictive performance. We use approximately 53,000 days of time-series Electronic Health Record (EHR) data taken from 10,000 ICU stays in the Multiparameter Intelligent Monitoring in Intensive Care IV (MIMIC-IV) dataset. Evaluating on a test holdout set of 10% of our data, we compare performance of our multi-task learning models to individually-trained deep networks that predict each component without parameter sharing. Model performance suggests that there is a small advantage to multi-task learning over unregularized networks, but no advantage compared to networks that employ regularization.
Date issued
2021-06Department
Massachusetts Institute of Technology. Department of Electrical Engineering and Computer SciencePublisher
Massachusetts Institute of Technology