The Limits of Analytics During Black Swan Events A Case Study of the Covid-19 Global Pandemic
Author(s)
Kaminski, Erez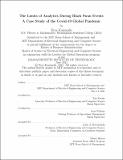
DownloadThesis PDF (1.438Mb)
Advisor
Kraska, Tim
Willems, Sean
Terms of use
Metadata
Show full item recordAbstract
During statistically unlikely events (Black Swan events) analytical models fail to provide their expected level of fidelity: their error can increase by several hundred percent. The modern economy is built on such analytical models, which are intended to provide useful results during routine conditions. All models eventually fail to provide the expected level of fidelity under extreme conditions. This thesis investigates the critical limitations of analytical methods during Black Swan events. Specifically, we study the space of possible model errors for statistical forecasting models and their respective implications for supply chain systems. We explore the forecast errors through numerical simulation and a real-world case study of a global manufacturing company experiencing the Covid-19 pandemic, a Black Swan event. We demonstrate that in some cases demand can shift by over 60%, leading to the bifurcation of the forecast error space, and resulting in an 500% increase in forecast error. This new regime causes supply chain planning systems to grind to a halt as existing inventory models become irrelevant. Such a drastic change in a company’s operational environment requires urgent action to ensure continued operations. For management to make correct decisions, it is critical for them to understand the limits of analytics during Black Swan events.
Date issued
2021-06Department
Massachusetts Institute of Technology. Department of Electrical Engineering and Computer Science; Sloan School of ManagementPublisher
Massachusetts Institute of Technology