Data-Efficient Offline Reinforcement Learning with Heterogeneous Agents
Author(s)
Alumootil, Varkey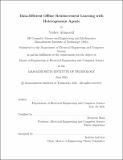
DownloadThesis PDF (1.225Mb)
Advisor
Shah, Devavrat
Terms of use
Metadata
Show full item recordAbstract
Performance of state-of-the art offline and model-based reinforcement learning (RL) algorithms deteriorates significantly when subjected to severe data scarcity and the presence of heterogeneous agents. In this work, we propose a model-based offline RL method to approach this setting. Using all available data from the various agents, we construct personalized simulators for each individual agent, which are then used to train RL policies. We do so by modeling the transition dynamics of the agents as a low rank tensor decomposition of latent factors associated with agents, states, and actions. We perform experiments on various benchmark environments and demonstrate improvement over existing offline approaches in the scarce data regime.
Date issued
2021-06Department
Massachusetts Institute of Technology. Department of Electrical Engineering and Computer SciencePublisher
Massachusetts Institute of Technology