Detecting incidents, accelerating dataset annotation, and estimating depth with multi-view invariants
Author(s)
Weber, Ethan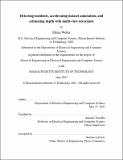
DownloadThesis PDF (71.57Mb)
Advisor
Torralba, Antonio
Terms of use
Metadata
Show full item recordAbstract
Computer vision has seen incredible growth since the introduction of large datasets, deep neural networks, and modern computing resources. Current algorithms can perform scene understanding, or the ability to understand and interpret the world through visual perception (e.g., images or videos). In this thesis, we push the boundaries of current scene understanding algorithms with three distinct projects. (1) In the first project, we address limitations of current algorithms to understand natural disasters, damage, and incidents through images. To do this, we create the Incidents Dataset, train a detection model, and present applications to identify incidents in social media streams to inform emergency responders during disaster relief situations. (2) In the second project, we address the issue of costly dataset construction and present a novel framework that reduces the cost of creating large-scale instance annotation datasets. (3) In the third and final project, we move to 3D scene understanding and present an intuitive technique to train monocular depth estimation networks by enforcing consistency of multi-view geometric invariants between image pairs observing the same scene or objects from the same category.
Date issued
2021-06Department
Massachusetts Institute of Technology. Department of Electrical Engineering and Computer SciencePublisher
Massachusetts Institute of Technology