Language Models Predict Drug Resistance from Complex Sequence Variation
Author(s)
Tso, Andy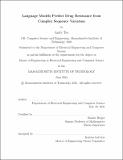
DownloadThesis PDF (10.31Mb)
Advisor
Berger, Bonnie
Terms of use
Metadata
Show full item recordAbstract
Mutation in viruses and bacteria presents a major barrier to the development of vaccines, antiviral drugs, and antibiotics. Recently, neural language models trained on viral protein sequence evolution have shown promise in their ability to predict viral escape mutations, potentially enabling more intelligent therapeutic design [6]. Hie et al.’s work puts forth the key conceptual advance that viral escape from human immunity occurs in the event of a mutation which simultaneously generates meaningful antigenic change while also preserving viral fitness. These ideas are analogous to the semantics and grammar of a language.
Theoretically, mutations that confer high semantic change while preserving high grammaticality may also be predictive of resistance to other types of evolutionary pressure as well. In this thesis, we show that language modeling of protein evolution can also predict mutations that confer drug resistance. We validate our language model predictions using known drug resistance mutations in HIV-1 protease and reverse transcriptase proteins and Escherichia coli beta-lactamase protein. Our results suggest a way to identify and potentially anticipate drug resistance mutations that generalizes across viruses and bacteria
Date issued
2021-06Department
Massachusetts Institute of Technology. Department of Electrical Engineering and Computer SciencePublisher
Massachusetts Institute of Technology