Validation, Calibration, and Uncertainty Quantification of the Wofost Crop Simulation Model
Author(s)
Kahraman, Sule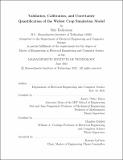
DownloadThesis PDF (4.411Mb)
Advisor
Hosoi, Anette “Peko”
Dahleh, Munther
Terms of use
Metadata
Show full item recordAbstract
The Digital Agriculture in Africa project [44] aims to unlock the agricultural potential in Africa and help farmers improve their crop productivity by enabling them to make more precise and timely decisions about crop management via better prediction tools. The absence of large, comprehensive, and structured agricultural data, however, limits the performance of these predictions. To address this problem, we propose a Data Platform that aggregates data from a variety of publicly available resources, processes them into reusable data formats, and augments the sparse data via synthetic data generation tools.
In this thesis, we focus on the synthetic generation of agricultural yield data via the WOFOST (WOrld FOod STudies) [20] crop simulation model. Through our validation, calibration, and uncertainty quantification steps, we seek to answer the following question: How can we reliably generate yield data for different regions of the world using simulation models?
Due to unavailability of large agricultural data from Africa, we chose to make use of data from the United States for the validation and calibration steps. Our empirical findings from the validation step demonstrated that the off-the-shelf usage of the WOFOST model, which was originally developed in Europe, may not be suitable for the agricultural studies in the United States, when the input parameters are not precise and accurate. This insight led us to perform the calibration step, where we discovered that the performance of the WOFOST model can be improved by estimating the correct crop parameters using evolutionary algorithms. Through our uncertainty quantification step, we shortlisted a number of input parameters that the model seems most sensitive to and developed a simpler but more tractable and effective model to WOFOST that has an analytical solution. Finally, we provided a quantitative analysis of how the uncertainty from the input parameters propagates through our proposed model to the generated data.
Date issued
2021-06Department
Massachusetts Institute of Technology. Department of Electrical Engineering and Computer SciencePublisher
Massachusetts Institute of Technology