Reducing Physician Burnout and Costs in Outpatient Healthcare Settings via Advanced Analytics
Author(s)
Escribe, Célia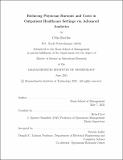
DownloadThesis PDF (1.977Mb)
Advisor
Levi, Retsef
Terms of use
Metadata
Show full item recordAbstract
National studies show that US primary care physicians are at high risk of burnout. Burnout has severe consequences for physicians, patients, and the healthcare system itself, which makes it one of the top priorities to be addressed by healthcare leaders today. In parallel, electronic health record systems (EHRs) have become ubiquitous in most practices, changing profoundly the work of primary care physicians, while offering at the same time new opportunities to analyze in a quantitative way various aspects of physicians’ work. This thesis offers actionable insights driven by data and analytics to address the previously mentioned key healthcare challenges.
Chapter 2 leverages advanced text analytics to identify work themes of primary care physicians related to inbox message management. A scalable methodology relying on a Latent Dirichlet Allocation model is developed to analyze physicians’ inbox work themes in great level of detail. This methodology could be used to implement appropriate workflow redesign in order to ensure that physicians spend most of their time on issues for which they have significant added value.
Chapter 3 employs a novel approach to examine team dynamics and their impact on physicians’ well-being. While many studies have tried to isolate factors related to physicians’ burnout, most of those studies are not scalable as they rely on self-evaluated surveys. This chapter addresses this question by providing a new quantitative methodology to analyze care team dynamics and structure through the integration of EHR data with social network modeling. Machine learning models are then developed to predict different dimensions of physicians’ well being using predictors related to team care dynamics and structure and work composition.
Chapter 4 finally develops a new modeling framework for a real-time appointment scheduling problem called the minimum peak appointment scheduling (MPAS) problem. While previous studies have applied existing algorithms from online bin packing to solve this problem, this modeling framework leverages unique aspects of appointment scheduling to further optimize scheduling decisions and reduce resource requirements. This chapter describes the first competitive online algorithm to the MPAS problem called the harmonic rematching (HR) algorithm, and proves that the HR algorithm has an asymptotic competitive ratio of 1.5.
Date issued
2021-06Department
Massachusetts Institute of Technology. Operations Research CenterPublisher
Massachusetts Institute of Technology