Data Driven Operations: From Algorithm Development to Experimental Design
Author(s)
Zhao, Jinglong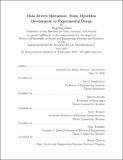
DownloadThesis PDF (2.496Mb)
Advisor
Simchi-Levi, David
Terms of use
Metadata
Show full item recordAbstract
Digital innovation has gained increasing attention in today's world. The explosion of data that is generated through modern marketplaces provides new opportunities to use data-driven tools to understand and optimize the marketplace operations. This dissertation studies various problems around the following two pillars of data-driven operations: optimization and econometrics.
In the first module we focus on optimization, in which we consider dynamic resource allocation problems under zero adaptivity. Dynamic resource allocation problems are omnipresent in modern business operations. In the revenue management setting, there are unreplenishable resources to allocate to heterogeneous consumer demands, immediately and irrevocably upon their arrivals. In such settings, zero adaptivity refers to a policy whose actions are independent of the remaining resources. Traditional revenue management literature has mainly focused on fully adaptive policies; and there is a gap between the provable effectiveness of adaptive policies in theory, and the applicability of non-adaptive policies in practice. We show that under different models of demand uncertainty, carefully designed non-adaptive policies may provably perform almost as well as the best fully adaptive counterparts.
In the second module we focus on econometrics, in which we consider experimental design problems. Experimental design is a widely adopted approach for firms to evaluate the effectiveness of their initiatives, by comparing the standard offering to a new initiative. Such a task is often challenging due to interference, both over time and across units. Traditional experimental design methods suffer from large variances of the estimators when accounting for interference; and practitioners have recognized that insufficient precision may lead to unreliable inference. We build the theoretical foundations to use optimization approach to maximize the precision when designing experiments.
Finally, we conclude with discussions of the limitations of the models and methods we have considered. We also provide practical suggestions to applied researchers and data scientists.
Date issued
2021-06Department
Massachusetts Institute of Technology. Institute for Data, Systems, and SocietyPublisher
Massachusetts Institute of Technology