Learning Robust Terrain-Aware Locomotion
Author(s)
Margolis, Gabriel B.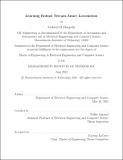
DownloadThesis PDF (22.45Mb)
Advisor
Agrawal, Pulkit
Terms of use
Metadata
Show full item recordAbstract
Today’s robotic quadruped systems can walk over a diverse set of natural and complex terrains. Approaches to locomotion based on model-based feedback control are robust to perturbations but cannot easily incorporate visual terrain information. Meanwhile, approaches to locomotion based on learning excel at associating visual sensory data with suitable control policies but often fail to generalize across the gap between simulation and deployment settings. This thesis proposes a trajectory-based abstraction for locomotion through which model-free and model-based control layers interface. This approach enables general visually guided locomotion while preserving robustness. We demonstrate that our proposed architecture allows the Mini Cheetah quadruped to match theoretical performance limits in a set of visual tasks. The robustness and practicality afforded by our approach are demonstrated through evaluation on hardware.
Date issued
2021-06Department
Massachusetts Institute of Technology. Department of Electrical Engineering and Computer SciencePublisher
Massachusetts Institute of Technology