Accounting for Computational Expenditures in Bayesian Experimental Design
Author(s)
Zheng, Sue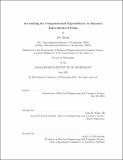
DownloadThesis PDF (6.006Mb)
Advisor
Fisher III, John W.
Terms of use
Metadata
Show full item recordAbstract
Many analysis problems involve the collection of data. The task of selecting the conditions for the data collection process is known as experimental design. The Bayesian optimal experimental design (BOED) formulation uses Bayesian inference to update beliefs after observing data and optimizes a utility function – most commonly mutual information – and is computationally challenging. Real-world problems entail complicated forward models that relate the data to the unknown quantities of interest. Identification of informative designs requires use of these models to assess the potential data provided under different experimental conditions. Furthermore, evaluation of information measures is generally intractable and estimation is often computationally expensive. This work focuses on computation as a resource constraint in experimental design and it appears in two very different ways.
The first relates to choices in the representation and how they influence the cost of experimental design. We explore this in the sensor planning problem for detecting special nuclear materials in cargo containers. We demonstrate the costs and benefits of modeling an additional physics phenomenon (i.e., Compton scattering) with respect to inference costs and information gain. While these results are specific to this problem, it demonstrates how consideration to the representation can lead to computational savings.
Secondly, we consider computational resources required in evaluating information measures. In this setting, we take an explicit treatment of the computational costs to develop an approach using a robust estimator that facilitates computation reuse between rounds of data collection and another that adaptively allocates computation towards targeted designs in a cost-aware manner. Importantly, this latter approach overtly trades off computation for performance, allowing one to strike their desired balance between the costs and benefits of experimental design and can be used in problems with a limited computational budget. We expect these approaches to broaden the application of Bayesian experimental design
Date issued
2021-06Department
Massachusetts Institute of Technology. Department of Electrical Engineering and Computer SciencePublisher
Massachusetts Institute of Technology