Essays on the Design of Online Marketplaces and Platforms
Author(s)
Holtz, David M.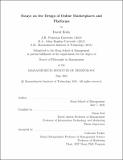
DownloadThesis PDF (11.89Mb)
Advisor
Aral, Sinan
Terms of use
Metadata
Show full item recordAbstract
This dissertation consists of three chapters that concern the design of online marketplaces and platforms. In Chapter 1, I estimate the impact of increasing the extent to which content recommendations are personalized by analyzing the results of a randomized experiment on approximately 900,000 Spotify users across seventeen countries. I find that increasing recommendation personalization increased the number of podcasts that Spotify users streamed, but also decreased the individual-level diversity of Spotify users’ podcast consumption and increased the dissimilarity between the podcast consumption patterns of different users across the population. In Chapter 2, I propose methods for obtaining unbiased estimates of the total average treatment effect (TATE) when conducting experiments in online marketplaces, and test the viability of said methods using a simulation built on top of scraped data from Airbnb. I find that blocked graph cluster randomization can reduce the bias of TATE estimates in online marketplaces by as much as 64.5%, however, this reduction in bias comes with a substantial increase in root-mean-square error (RMSE). I also find that fractional neighborhood treatment response (FNTR) exposure models and inverse probability-weighted estimators have the potential to further reduce bias, depending on the choice of FNTR threshold. In Chapter 3, I conduct two large-scale meta-experiments on Airbnb in an attempt to estimate the actual magnitude of bias in TATE estimates from marketplace interference. In both meta-experiments, some Airbnb listings are assigned to experiment conditions at the individual-level, whereas others are assigned to experiment conditions at the level of clusters of listings that are likely to substitute for one another. The two meta-experiments measure the impact of two different pricing-related interventions on Airbnb: a change to Airbnb’s fee policy, and a change to the pricing algorithm that Airbnb uses to recommend prices to sellers. Results from the fee policy meta-experiment reveal that at least 32.60% of the treatment effect estimate in the Bernoulli-randomized meta-experiment arm is due to interference bias. Results from the pricing algorithm meta-experiment highlight the difficulty of detecting interference bias when treatment interventions require intention-to-treat analysis.
Date issued
2021-06Department
Sloan School of ManagementPublisher
Massachusetts Institute of Technology