Predictive Modeling and Optimization of Autoinjector Manufacturing
Author(s)
DeLuke, Levi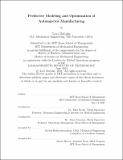
DownloadThesis PDF (16.40Mb)
Advisor
Roche, Ellen
Jónasson, Jónas
Terms of use
Metadata
Show full item recordAbstract
The manufacture of drug delivery devices requires the assembly of multiple device components with the corresponding drug products, often with an abundance of data being collected at each assembly stage. This data represents an underutilized resource for process improvement, due to disparate data sources residing in separate organizations and within different source databases for each individual component or process stage. This research aims to improve the manufacture of drug delivery devices using upstream component and process data to improve patient experience and the performance of assembled devices. An interpretable predictive modeling framework is developed to identify sources of subcomponent and process variability that are predictive of final lot performance. Differences in predictive accuracy across products, user demographics, geography, and with different sources of component data are determined and used to inform ongoing data management and process improvement recommendations. The predictive modeling framework is then applied towards the creation of a production planning tool to predict and optimize the pairing of subcomponent batches to best meet final product specifications and improve performance. A simulation is used to estimate the impact of the proposed pairing strategies, resulting in an estimated 35-45 percent reduction in variability of a final product parameter over existing methods. The initial case study on a single product family is generalized to a modeling framework with broader applicability.
Date issued
2021-06Department
Massachusetts Institute of Technology. Department of Mechanical Engineering; Sloan School of ManagementPublisher
Massachusetts Institute of Technology