A System for High-Throughput Materials Exploration Driven by Machine Learning
Author(s)
Siemenn, Alexander E.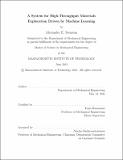
DownloadThesis PDF (28.07Mb)
Advisor
Buonassisi, Tonio
Terms of use
Metadata
Show full item recordAbstract
Functional materials have vast and high-dimensional compositions spaces which make discovering optimized compositions intractable with conventional synthesis tools. Conventional experimental methods of exploring material composition spaces are slow and resource intensive due to being manual processes and requiring trial-and-error experimentation. Thus, the question is posed: How can we design an optimized functional material from this highly dimensional and vast composition space such that it has a high performance for a given application?
In this thesis, machine learning algorithms are integrated into novel, high-throughput synthesis hardware to accelerate the rate of material composition exploration by 10000x relative to these conventional methods. First, a novel inkjet droplet deposition hardware system is constructed to generate arrays of unique functional material compositions in the form of droplets using fluid mechanics and motor control theory. Second, computer vision and Bayesian optimization machine learning algorithms are integrated into the droplet synthesis loop to autonomously discover synthesis conditions that generate optimized droplets without any intervention of a domain expert. Third, mulitphysics models are developed to simulate the performance of functional material devices within the gamut of environmental conditions without having to run expensive laboratory experiments. The culmination of these three processes developed in this master's thesis provide validated methods for driving high-throughput, low-cost materials exploration and optimization to be further explored in my doctoral thesis.
Date issued
2021-06Department
Massachusetts Institute of Technology. Department of Mechanical EngineeringPublisher
Massachusetts Institute of Technology