The Application of Double Machine Learning Onto Genomics Data Associated with Amyotrophic Lateral Sclerosis
Author(s)
Wang, Crystal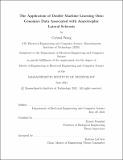
DownloadThesis PDF (1.546Mb)
Advisor
Fraenkel, Ernest
Terms of use
Metadata
Show full item recordAbstract
Finding causal relationships between a dataset and an observed outcome is especially important when there is potential for meaningful interventions. One such area of focus is a biological setting, where there are many opportunities for diagnosis, prevention, and treatment research. Amyotrophic Lateral Sclerosis (ALS) is a progressive neurodegenerative disease for which which there is no cure and relatively little is known about what causes the disease. Previous work has shown certain genes to be associated with ALS and previous work have used machine learning to try and determine the causal features of ALS. In this thesis we experiment with Double Machine Learning [8] to find causal features of ALS. We apply this method on both synthetic and real datasets that are associated with ALS and explain the advantages and shortcomings of this methodology on genetics data where correlation is present.
Date issued
2021-06Department
Massachusetts Institute of Technology. Department of Electrical Engineering and Computer SciencePublisher
Massachusetts Institute of Technology