Compositional Models for Few Shot Sequence Learning
Author(s)
Akyurek, Ekin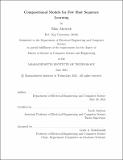
DownloadThesis PDF (1.285Mb)
Advisor
Andreas, Jacob
Terms of use
Metadata
Show full item recordAbstract
Flexible neural sequence models outperform grammar- and automaton-based counterparts on a variety of tasks. However, neural models perform poorly in settings requiring compositional generalization beyond the training data—particularly to rare or unseen subsequences. Past work has found symbolic scaffolding (e.g. grammars or automata) essential in these settings. We describe two simpler and more general modeling approaches that enable a large category of compositional generalizations without appeal to latent symbolic structure. The first is a data augmentation scheme called R&R, built from two components: recombination of original training examples via a prototype-based generative model and esampling of generated examples to encourage extrapolation. Training an ordinary neural sequence model on a dataset augmented with recombined and resampled examples significantly improves generalization in two language processing problems—instruction following SCAN and morphological analysis SIGMORPHON (2018)—where R&R enables learning of new constructions and tenses from as few as eight initial examples. The second is a lexical translation mechanism for neural sequence modeling. Previous work shows that many failures of systematic generalization arise from neural models' inability to disentangle lexical phenomena from syntactic ones. To address this, we augment neural decoders with a lexical translation mechanism that generalizes existing copy mechanisms to incorporate learned, decontextualized, token-level translation rules. We describe how to initialize this mechanism using a variety of lexicon learning algorithms, and show that it improves systematic generalization on a diverse set of sequence modeling tasks drawn from cognitive science, logical semantics, and machine translation.
Date issued
2021-06Department
Massachusetts Institute of Technology. Department of Electrical Engineering and Computer SciencePublisher
Massachusetts Institute of Technology