Mathematical and Computational Foundations to Enable Predictive Digital Twins at Scale
Author(s)
Kapteyn, Michael G.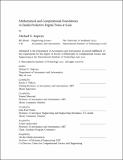
DownloadThesis PDF (22.19Mb)
Advisor
Willcox, Karen E.
Terms of use
Metadata
Show full item recordAbstract
A digital twin is a computational model that evolves over time to persistently represent a unique physical asset. Digital twins underpin intelligent automation by enabling asset-specific analysis and data-driven decision-making. Although the promise of digital twins is well established, state-of-the-art digital twins are typically bespoke, one-off implementations that require considerable expertise and deployment resources. This thesis develops mathematical and computational foundations to support the transition from this custom implementation phase toward accessible and robust digital twins at scale.
First, a unified mathematical foundation for digital twins is established. A mathematical abstraction of a digital twin and its associated physical asset is presented. This abstraction is then developed into a probabilistic graphical model describing the evolution of the coupled system. This model affords a unified treatment of all the aspects of a digital twin and can span the entire asset lifecycle. While mathematically rigorous, the model is flexible and extensible to enable application in a wide range of application areas.
Building on this mathematical foundation, scalable computational methodologies are developed to enable asset-specific physics-based models to be incorporated into a digital twin. A central element of the proposed approach is a library of component-based reduced-order models derived from high-fidelity simulations of the asset in various states. The component-based approach scales efficiently to complex systems and provides a flexible and expressive framework for model adaptation—both critical features in the digital twin context. A methodology is proposed for combining these physics-based models with interpretable machine learning techniques in order to determine which observational data are most informative, and how these data can be fused within an interpretable classifier. This classifier can be deployed online to enable dynamic data-driven updating of the digital twin.
The proposed methodologies are demonstrated through the creation, calibration, and deployment of a structural digital twin for a custom-built 12ft wingspan unmanned aerial vehicle. In flight, the digital twin assimilates sensor data to update its internal structural models in response to damage or degradation. The dynamically updated digital twin provides rapid computational analysis of the vehicle’s structural health, which in turn enables intelligent self-aware decision-making.
Date issued
2021-06Department
Massachusetts Institute of Technology. Department of Aeronautics and AstronauticsPublisher
Massachusetts Institute of Technology