Enhancing Corporate Strategy Using Data-Driven Business Growth Decisions
Author(s)
Nepsky, Patrick A.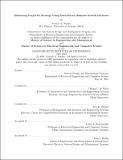
DownloadThesis PDF (2.122Mb)
Advisor
Welsch, Roy E.
de Weck, Olivier L.
Terms of use
Metadata
Show full item recordAbstract
Corporate strategy – the process of formulating and achieving company objectives – is a broad area involving many types of complex decisions. This thesis explores a data-driven approach to executing business growth decisions. Specifically, the thesis provides a machine-learning framework that can be used to predict outcomes of two major corporate growth decisions: investment into internal research and development (R&D) projects, and acquisitions of external early-stage ventures. Historically, these two growth decisions have primarily been made by small teams of subject matter experts, and have not been thoroughly explored using modern machine learning methodologies. The prediction problems in this thesis are framed as binary classification schemes, and the outcomes are predicted using a custom feature set generated from structured databases. The initial results show significantly better-than-random performance for both growth prediction problems, with Area Under the Curve (AUC) of model Receiver Operating Characteristics (ROC) reaching as high as 0.7–0.8. This performance is consistent across a range of different model architectures. A portfolio simulation suggests that the binary outcome prediction may be sufficient information in order to generate positive financial returns. Future work should explore more complex system models, different and/or multi-class prediction targets, and real-time data fusion frameworks to incorporate the model recommendations into corporate strategy workflow.
Date issued
2021-06Department
Massachusetts Institute of Technology. Department of Electrical Engineering and Computer Science; System Design and Management Program.Publisher
Massachusetts Institute of Technology