The Detection and Characterization of Severe Features in Colonoscopy Videos Using Combined Segmentation and Classification Models
Author(s)
Wang, Yi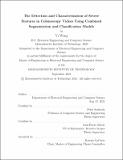
DownloadThesis PDF (4.056Mb)
Advisor
Szolovits, Peter
Schott, Jean-Pierre
Terms of use
Metadata
Show full item recordAbstract
Inflammatory Bowel Disease is a chronic disease that requires regular monitoring procedures, such as colonoscopy. Assessing disease severity through endoscopy is critical to determining therapeutic responses in IBD, but its use in clinical practice is limited by the requirement for experienced human reviewers. In recent development, artificial intelligence is used to evaluate endoscopic disease severity based on colonoscopy images. However, due to the variability in disease phenotypes, the nonrigid nature of objects, and clinical artifacts, very few groups have studied image or video segmentation for colonoscopy videos, and the existing deep learning systems showed low transparency and traceability. We propose a feature-based model that breaks down the problem into smaller components and combines segmentation and classification models to characterize IBD features and predict disease severity for frame-level and clip-level data. Our combined segmentation and classification models had an average accuracy of 90% for the detection of severe IBD features such as ulcerations and erosions. This thesis was completed at Iterative Scopes, a Boston startup working on bringing precision medicine and technology to gastroenterology.
Date issued
2021-09Department
Massachusetts Institute of Technology. Department of Electrical Engineering and Computer SciencePublisher
Massachusetts Institute of Technology