Sampling-Based Robot Task and Motion Planning in the Real World
Author(s)
Garrett, Caelan Reed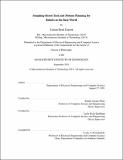
DownloadThesis PDF (69.05Mb)
Advisor
Lozano-Pérez, Tomás
Kaelbling, Leslie Pack
Terms of use
Metadata
Show full item recordAbstract
We seek to program a robot to autonomously complete complex tasks in a variety of real-world settings involving different environments, objects, manipulation skills, degrees of observability, initial states, and goal objectives. In order to successfully generalize across these settings, we take a model-based approach to building the robot’s policy, which enables it to reason about the effects of it executing different sequences of parameterized manipulation skills. Specifically, we introduce a general-purpose hybrid planning framework that uses streams, modules that encode sampling procedures, to generate continuous parameter-value candidates. We present several domain-independent algorithms that efficiently combine streams in order to solve for parameter values that jointly satisfy the constraints necessary for a sequence of skills to achieve the goal. Each stream can be either engineered to perform a standard robotics subroutine, like inverse kinematics and collision checking, or learned from data to capture difficult-to-model behaviors, such as pouring, scooping, and grasping. Streams are also able to represent probabilistic inference operations, which enables our framework to plan in belief space and intentionally select actions that reduce the robot’s uncertainty about the unknown world. Throughout this thesis, we demonstrate the generality of our approach by applying it to several real-world tabletop, kitchen, and construction tasks and show that it can even be effective in settings involving objects that the robot has never seen before.
Date issued
2021-09Department
Massachusetts Institute of Technology. Department of Electrical Engineering and Computer SciencePublisher
Massachusetts Institute of Technology