Implementing CAST and Designing the STAMP-Enhanced Learning And Reporting System
Author(s)
Wong, Lawrence Man Kit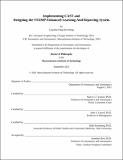
DownloadThesis PDF (23.74Mb)
Advisor
Leveson, Nancy G.
Carroll, John S.
Rosenberg, Beth
Terms of use
Metadata
Show full item recordAbstract
The safety performance of healthcare is concerning. Around 6% of patients are impacted by preventable harm. Adverse events recur because incident reporting systems (IRSs) are not effective and causes are not addressed. In-depth incident analyses, in particular, often focus on frontline human error and are blame-laden. All IRS functions, from data collection to learning dissemination, need to be improved. CAST (based on a state-of-the-art accident causality model, STAMP) is a more effective analysis technique, but its application in healthcare is hindered by time and knowledge constraints. This work investigated how CAST can be introduced and what features are required in a more effective IRS.
Seven enhancements were made, encompassing methodological refinement, reference materials, templates, and training. The enhancements seek to make CAST applications more efficient and consistent for novices. For evaluation, a hospital safety team was trained and analyzed an incident with CAST. The analysis not only identified the unsafe actions by frontline staff but also the underlying reasons. The departmental management’s unsafe decisions and their underlying reasons were identified as well. The proposed safety interventions had broad system coverage, the potential for hazard elimination and could prevent dissimilar incidents. More learning was gained than with the conventional technique. Moreover, the analysis—produced with less time, training and without the guidance of a safety science expert—was at least comparable to, if not better than, other CAST analyses done by novices without the enhancements. Self-reported attitude agreement suggests a paradigm change may have been made. However, self-confidence in analysis abilities did not differ substantially, suggesting the training program should be revised to reconcile the mismatch with the improved analysis performance.
For broader IRS improvements, a conceptual design for the STAMP-Enhanced Learning And Reporting (STELAR) system was created. It focuses on improving the interdependencies between IRS functions and with external safety information sources.
CAST can be feasibly learned and applied in healthcare; IRSs can be improved by designing it holistically. This work advances the goal to research, develop, and apply systems engineering tools in healthcare and contributes to a safer healthcare system—by enabling effective safety learning with a systems approach.
Date issued
2021-09Department
Massachusetts Institute of Technology. Department of Aeronautics and AstronauticsPublisher
Massachusetts Institute of Technology