Image registration and bias evaluation for a COVID-19 pulmonary X-ray severity (PXS) score prediction algorithm
Author(s)
Agarwal, Vibha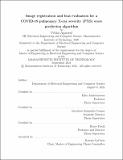
DownloadThesis PDF (28.87Mb)
Advisor
Adalsteinsson, Elfar
Kalpathy-Cramer, Jayashree
Fischl, Bruce
Terms of use
Metadata
Show full item recordAbstract
As COVID-19 spreads, it is increasingly important to track disease trajectory in order to provide better care for patients. Analyzing chest x-rays (CXRs) is one method used by radiologists to assess disease severity, but manual interpretation is time-consuming and subject to inter- and intra-rater variability. One study has used a Siamese neural network to predict numerical COVID-19 pulmonary disease severity scores [19], but because CXRs from the same patient tend to have differences in positioning and acquisition unrelated to disease progression, image registration can be used to standardize the CXRs to improve longitudinal comparison. In this study, we show that affine image registration using Voxelmorph [3] has the potential to improve the prediction of longitudinal change in COVID-19 severity. Additionally, external generalization is a challenging problem for medical AI, and a model used in healthcare settings must be free of bias in order to be clinically valid. To this end, we analyze the performance of the Siamese prediction model on an external dataset and show that its predictions correlate with expert disease severity labels, and that it performs similarly for different demographic groups (age, sex, BMI, and international location). These preliminary results suggest that the model may be a reliable and equitable way, among the subgroups evaluated, to quantify disease severity labels.
Date issued
2021-09Department
Massachusetts Institute of Technology. Department of Electrical Engineering and Computer SciencePublisher
Massachusetts Institute of Technology