Probabilistic Oil and Gas Production Forecasting using Machine Learning
Author(s)
Andrais, Robert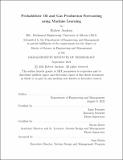
DownloadThesis PDF (6.794Mb)
Advisor
Fournier, Aimé
Moser, Bryan
Terms of use
Metadata
Show full item recordAbstract
This thesis improves oil- and gas-well profitability by quantifying the uncertainty of the production-forecasting process, using probabilistic machine learning (ML) techniques. A Bayesian Neural Network successfully modelled a complex shale gas reservoir system (Eagle Ford), generating a production forecast with 5% mean absolute percent error. This result is 10%–35% more accurate than traditional decline curve analysis. These forecasts also quantified the epistemic and aleatory uncertainties, providing plausible probabilistic P10 and P90 values. This range provides analysts with the capability of making informed strategic decisions that consider risk. Next, the model was applied to predict reserves (estimated ultimate recovery) and the underlying reservoir quality. These predictions were combined with unsupervised learning techniques (Gaussian Mixture Modelling), creating gas and oil sweet-spot maps. Finally, this workflow’s robustness was demonstrated by artificially reducing data by 93%; indeed, the algorithm could reproduce the full-dataset results with a 71%–91% Pearson correlation, despite this reduction. Supporting this workflow creation is an evaluation of relevant research, data processing, feature engineering, documentation of the probabilistic ML structure, and discussion of model performance using systems analysis.
Date issued
2021-09Department
System Design and Management Program.Publisher
Massachusetts Institute of Technology