Benchmarking the Performance of Bayesian Optimization across Multiple Experimental Materials Science Domains
Author(s)
Liang, Qiaohao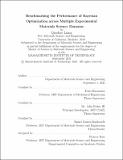
DownloadThesis PDF (9.373Mb)
Advisor
Buonassisi, Tonio
Fisher III, John
Gomez-Bombarelli, Rafael
Terms of use
Metadata
Show full item recordAbstract
Traditionally, experimental materials optimization has used design of experiments or intuition, combined with in-depth characterization. While these methods have obtained success over the years, they are facing increasing challenges today in the face of complex aggregated systems with larger design spaces. The materials objectives for these systems, e.g. environmental stability of solar cells or toughness of 3D printed mechanical structures, are typically costly to simulate and slow to experimentally evaluate. The need to shorten lab-to-market time of functional materials has inspired the use of machine learning and automation in materials optimization. Active learning algorithms, such as Bayesian Optimization (BO), have been leveraged for guiding autonomous high-throughput experimentation (HTE) systems. There have been individual studies successfully applying BO in experimental materials optimization, yet very few evaluated the performance of BO as a general optimization algorithm across a broad range of materials science domains.
In this work, we benchmark the performance of BO algorithms with a collection of surrogate model and acquisition function pairs across five diverse experimental materials systems, including carbon nanotube polymer blends, silver nanoparticles, lead-halide perovskites, as well as additively manufactured polymer structures and shapes. By defining acceleration and enhancement performance metrics as general materials optimization objectives, we find that for surrogate model selection, Gaussian Process (GP) with anisotropic kernels (automatic relevance detection, ARD) and Random Forests (RF) have comparable performance and both outperform the commonly used GP without ARD. We discuss the implicit distributional assumptions of RF and GP, and the benefits of using GP with anisotropic kernels in detail. We provide practical insights for experimentalists on surrogate model selection of BO during materials optimization campaigns.
Date issued
2021-09Department
Massachusetts Institute of Technology. Department of Materials Science and EngineeringPublisher
Massachusetts Institute of Technology