Memristor-based AI Hardware for Reliable and Reconfigurable Neuromorphic Computing
Author(s)
Choi, Chanyeol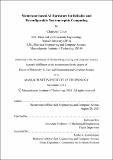
DownloadThesis PDF (5.613Mb)
Advisor
Kim, Jeehwan
Terms of use
Metadata
Show full item recordAbstract
In the field of artificial intelligence hardware, a memristor has been proposed as an artificial synapse for creating neuromorphic computer applications. Changes in weight values in the form of conductance must be identifiable and uniform to train a neural network in memristor arrays. Because of the high mobility of metal ions in the Si switching medium, an electrochemical metallization (ECM) memory has shown a high analogue switching capacity. However, switching unpredictability is caused by the extreme stochasticity of ion transport. I demonstrated a Si memristor with alloyed conduction channels that works dependably and enables large-scale crossbar array deployment. In addition, heterogeneously integrated neuromorphic chips have been developed to allow physically reconfigurable neuromorphic computing. This thesis examines alloyed metal-based silicon memristors and stackable neuromorphic chips with heterogeneous integration for reliable and reconfigurable neuromorphic computing.
Date issued
2021-09Department
Massachusetts Institute of Technology. Department of Electrical Engineering and Computer SciencePublisher
Massachusetts Institute of Technology