Machine Learning and Variational Algorithms for Lattice Field Theory
Author(s)
Kanwar, Gurtej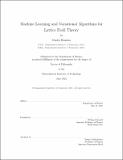
DownloadThesis PDF (3.774Mb)
Advisor
Detmold, William
Terms of use
Metadata
Show full item recordAbstract
Discretizing fields on a spacetime lattice is the only known general and non-perturbative regulator for quantum field theory. The lattice formulation has, for example, played an important role in predicting properties of QCD in the strongly coupled regime, where perturbative methods break down. To recover information about continuum physics, parameters defining the lattice theory must be tuned toward criticality. However, Markov chain Monte Carlo (MCMC) methods commonly used to evaluate the lattice-regularized path integral suffer from critical slowing down in this limit, restricting the precision of continuum extrapolations. Further difficulties arise when computing the energies and interactions of physical states by measuring correlation functions of operators widely separated in spacetime: for most correlation functions, an exponentially severe signal-to-noise problem is encountered as the operators are taken to be widely separated, limiting the precision of calculations.
This dissertation introduces two new techniques to address these issues. First, we define a novel MCMC algorithm based on generative flow-based models. Such models utilize machine learning methods to describe efficient approximate samplers for distributions of interest. Independently drawn flow-based samples are then used as proposals in an asymptotically exact Metropolis-Hastings Markov chain. We also construct models that flexibly parameterize families of distributions while capturing symmetries of interest, including translational and gauge symmetries. By variationally optimizing the distribution selected from these families, one can maximize the efficiency of flow-based MCMC. We secondly introduce an approach to 'deform' Monte Carlo estimators based on contour deformations applied to the domain of the path integral. The deformed estimators associated with an observable give equivalent unbiased measurements of that observable, but generically have different variances. We define families of deformed manifolds for lattice gauge theories and introduce methods to efficiently optimize the choice of manifold (the 'observifold') so that the variance of the associated deformed observable is minimized. Finally, we demonstrate that flow-based MCMC can mitigate critical slowing down and observifolds can exponentially reduce variance in proof-of-principle applications to scalar phi^4 theory and U(1) and SU(N) lattice gauge theories.
Date issued
2021-06Department
Massachusetts Institute of Technology. Department of PhysicsPublisher
Massachusetts Institute of Technology