Structural Priors for Active Learning on Robots
Author(s)
Brand, Isaiah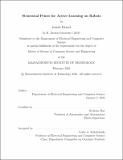
DownloadThesis PDF (14.21Mb)
Advisor
Roy, Nicholas
Terms of use
Metadata
Show full item recordAbstract
A primary hindrance to neural networks in robotic applications is data efficiency; collecting data on a real robot is slow and expensive. Active learning, in which the learner chooses the data that will best accelerate learning, has been shown to reduce data requirements in machine learning and statistics applications, but has seen limited application to real robots. This thesis leverages Bayesian Active Learning in robotic domains, and proposes two novel extensions that further improve data efficiency by exploiting the structure inherent to most robotics applications. First, we introduce active learning of Abstract Plan Feasibility (APF) --- the likelihood that a plan proceeds as expected when executed on the robot. By incorporating the learned APF model into the active learning loop, we significant improve data efficiency. This approach enables a real 7DOF Panda robot arm to learn a neural network estimator of APF in a block stacking domain after only 400 experiments. For comparison, state-of-the-art model learning methods require thousands or millions of interactions in similar domains. We show that the learned APF estimator significantly improves planning for downstream tasks. Second, we incorporate the notion of objects --- a structure present in many robotic domains --- into the active learning framework. We develop an object-factored dynamics model, which allows the robot to separate uncertainty about individual objects and the global dynamics. When paired with Bayesian active learning, the object-factored dynamics model allows the robot to actively learn about novel objects, without adjusting the global dynamics. We evaluate this approach in simulated block stacking and ball throwing environments.
Date issued
2022-02Department
Massachusetts Institute of Technology. Department of Electrical Engineering and Computer SciencePublisher
Massachusetts Institute of Technology