Improving Autonomous Navigation and Estimation in Novel Environments
Author(s)
Liu, Katherine Y.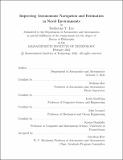
DownloadThesis PDF (18.71Mb)
Advisor
Roy, Nicholas
Terms of use
Metadata
Show full item recordAbstract
Efficient autonomous navigation in novel environments is crucial to enable embodied agents to reach more sophisticated levels of autonomy. We are interested in improving autonomous navigation and estimation in unknown environments of vehicles carrying lightweight electro-optical sensor payloads. Due to sensing limitations, in non-trivial novel environments much of the geometric structure of the world has not yet been observed, leading to significant geometric ambiguity. Although collecting additional geometric information can reduce ambiguity, doing so is often at odds with the objectives of the mission. We propose to combine object-level semantic information and geometric information to tractably improve both navigation and estimation.
In this thesis, we present three contributions towards improving autonomous navigation in novel environments. We first improve navigation efficiency in novel environments by encoding useful navigation behaviors in a sampling distribution informed by partial occupancy and object-level maps. Recognizing that object-level estimation is challenging under the limited viewpoints available while navigating efficiently, we also develop two methods of building object-level representations online. In our second contribution, we improve the view-point efficiency of object-level SLAM with ellipsoid representations by introducing an additional texture measurement and semantic class shape prior. Finally, in our third contribution, we propose a novel method of deeply learned 3D object estimation that utilizes indirect image-space annotations and intra-class shape consistency to enable 3D object estimation from a single RGB image.
Date issued
2022-02Department
Massachusetts Institute of Technology. Department of Aeronautics and AstronauticsPublisher
Massachusetts Institute of Technology