Physics-assisted machine learning for X-ray imaging
Author(s)
Guo, Zhen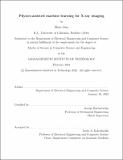
DownloadThesis PDF (16.28Mb)
Advisor
Barbastathis, George
Terms of use
Metadata
Show full item recordAbstract
X-ray imaging is capable of imaging the interior of objects in two and three dimensions non-invasively, with applications in biomedical imaging, materials study, electronic inspection, and other fields. The reconstruction process can be an ill-conditioned inverse problem, requiring regularization to obtain satisfactory reconstructions. Recently, deep learning has been adopted for 2D and 3D reconstruction. Unlike iterative algorithms which require a distribution that is known a priori, deep reconstruction networks can learn a prior distribution through sampling the statistical properties of the training distributions. In this thesis, we develop a physics-assisted machine learning algorithm, a two-step algorithm for 2D and 3D reconstruction. The 2D case is studied in the context of randomized probe imaging to retrieve quantitative phase distribution using deep k-learning framework, and 3D case is under X-ray tomography to retrieve the structure of integrated circuit via physics-assisted generative network. In contrast to previous efforts, our physics-assisted machine learning algorithm utilizes iterative approximants derived from the physical measurements to regularize the reconstruction with both known physical prior and the learned priors. The advantages of using learned priors from machine learning in X-ray imaging may further enable low-photon nanoscale imaging. Note that part of this thesis has been previously reported [1, 2].
Date issued
2022-02Department
Massachusetts Institute of Technology. Department of Electrical Engineering and Computer SciencePublisher
Massachusetts Institute of Technology