Deep Learning for the KamLAND-Zen Search for 0𝜈𝛽𝛽
Author(s)
Fraker, Suzannah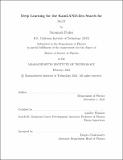
DownloadThesis PDF (10.43Mb)
Advisor
Winslow, Lindley
Terms of use
Metadata
Show full item recordAbstract
Neutrinoless double beta decay (0𝜈𝛽𝛽) is a major interest in neutrino physics. Discovery of 0𝜈𝛽𝛽 would demonstrate that neutrinos are Majorana fermions and that lepton number is not a symmetry of nature, thus providing a possible explanation for the observed matter-antimatter asymmetry of the universe. KamLAND-Zen is a leading search for 0𝜈𝛽𝛽, having placed the most stringent limit on its half-life at [formula] at 90% C.L. in ¹³⁶Xe. The next phase of KamLAND-Zen is currently running and will place even more stringent limits on the half-life. The sensitivity of KamLAND-Zen is primarily limited by backgrounds, including the muon spallation background ¹⁰C. We present a machine learning algorithm based on a convolutional neural network (CNN) that is able to separate ¹⁰C events from 136Xe events in Monte Carlo simulated data. With a typical kiloton-scale detector configuration like the KamLAND-Zen detector, we find that the algorithm is capable of identifying 61.6% of the ¹⁰C at 90% signal acceptance. The algorithm is independent of vertex and energy reconstruction, so it is complementary to current methods and can be expanded to other background sources.
Date issued
2022-02Department
Massachusetts Institute of Technology. Department of PhysicsPublisher
Massachusetts Institute of Technology