Wireless Sensing with Machine Learning: Through-Wall Vision & Contactless Health Monitoring
Author(s)
Zhao, Mingmin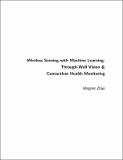
DownloadThesis PDF (63.57Mb)
Advisor
Katabi, Dina
Terms of use
Metadata
Show full item recordAbstract
There is significant interest in technologies that can sense people and monitor their health with minimal overhead. Existing solutions typically require people to wear different sensors and devices on their bodies. This thesis demonstrates how we can use wireless signals and machine learning to sense people without any physical contact with their bodies. We develop novel radio sensors that sit in the background like a Wi-Fi router. Our sensors however analyze the surrounding radio signals using novel machine learning algorithms to monitor people’s movements and activities, assess their vital signs, learn their sleep and sleep stages, and recognize their emotions. Since wireless sensors traverse walls, our sensors can deliver all of these functions through walls and occlusions.
The key challenge in delivering the above contributions is that radio signals interact with people and the environment in complex ways, resulting in an underdetermined mapping that varies across time and space. To address this problem, this dissertation adopts a data-driven approach and develops custom machine learning models that operate on radio signals. Developing such models requires technical innovations to address unique challenges due to the specularity of radio signals in the frequencies of interest, multipath reflections in indoor environments, high data rates and computation complexity, and the lack of training data and the difficulty in annotating radio signals. Our work addresses these challenges and enables two new capabilities: through-wall tracking of the human pose and contactless health monitoring.
Date issued
2022-02Department
Massachusetts Institute of Technology. Department of Electrical Engineering and Computer SciencePublisher
Massachusetts Institute of Technology