Improving Labor Market Design to Reduce Labor Abuse in the Global Supply Chain in Southeast Asia
Author(s)
Liu, Boyu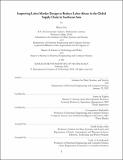
DownloadThesis PDF (3.336Mb)
Advisor
de Zegher, Joann
Terms of use
Metadata
Show full item recordAbstract
Forced labor and labor abuse have become a growing concern in the global supply chain. Evidence suggest that more than 25 million people are victims of forced labor, and that much of this problem stem from the recruitment process. In collaboration with the Issara Institute (Issara), a non-profit organization based in the US and Thailand, this work aims to improve the above issue in two parts. First, we evaluate the causal relationship between inefficiency in labor recruitment and labor abuse outcomes to provide evidence-based policy suggestion. Second, we design an algorithm of joint matching and learning for the recruitment platform built by Issara, named "Golden Dreams", that aims to make it easier for workers and recruiters to find suitable matches, while using data generated by this process to estimate fair labor practices by employers. Our goal is to create employer ratings that are truth-revealing to help workers make more informed choices, and help employers meet their labor demands faster and mitigate labor risk by monitoring their labor practices on the frontline.
Leveraging 2018-2020 datasets on Myanmar-Thailand labor recruitment and worker-reported abuses, we find that an inability to efficiently alleviate labor shortages significantly worsens workerreported abuses; an increase of one standard deviation in low-skilled labor shortages leads to a 34.5% or higher increase in worker-reported abuse in the following 2-4 weeks. Labor markets that are stressed are also simultaneously more prone to unexpected shortages and abuse. Reducing frictions in recruitment, and strengthening worker reporting mechanisms that provide near-real-time information about workplace labor abuse, are important avenues to eliminating forced labor.
As such, we collaborate with Issara on the design of a labor market to address this friction. The matching while learning part builds upon existing literature in the intersection of computer science and economics. The traditional market design literature assumes known preferences and perfect information, and the classical multi-armed bandits literature does not deal with market settings with collision of preferences and resource constraints. To develop a joint algorithm that satisfy standard axioms in a market setting, yet able to learn from historical data and leverage this learning with uncertainty to inform future actions, require an interdisciplinary approach. We propose such a combined approach. We then discuss practical considerations when putting it into practice as well as policy and social concerns.
Date issued
2022-02Department
Massachusetts Institute of Technology. Department of Electrical Engineering and Computer Science; Massachusetts Institute of Technology. Institute for Data, Systems, and SocietyPublisher
Massachusetts Institute of Technology