A Machine Learning Approach for Understanding and Discovering Topological Materials
Author(s)
Ma, Andrew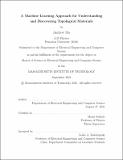
DownloadThesis PDF (3.167Mb)
Advisor
Soljačić, Marin
Terms of use
Metadata
Show full item recordAbstract
Topological materials are of significant interest for both basic science and next-generation technological applications due to their unconventional electronic properties. The majority of currently-known topological materials have been discovered using methods that involve symmetry-based analysis of the quantum mechanical wavefunction. Here we use machine learning to develop a heuristic chemical rule, which diagnoses whether a material is topological using only its chemical formula. It is based on a notion that we term topogivity, which is a learned numerical value for each element that loosely captures the tendency of an element to form topological materials. Topogivities provide chemical insights for understanding topological materials. We implement a high-throughput procedure for discovering topological materials that are not diagnosable by symmetry indicators. The procedure is based on heuristic rule prediction followed by ab initio validation. The concept of topogivity represents a fundamentally new approach to the study of topological materials, and opens up new directions of research at the intersection of chemistry, machine learning, and band topology.
Date issued
2021-09Department
Massachusetts Institute of Technology. Department of Electrical Engineering and Computer SciencePublisher
Massachusetts Institute of Technology