Machine Learning Methods for Image-based Personalized Cancer Screening
Author(s)
Yala, Adam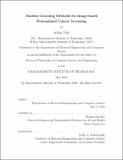
DownloadThesis PDF (16.55Mb)
Advisor
Barzilay, Regina
Terms of use
Metadata
Show full item recordAbstract
While AI has the potential to transform patient care, the development of equitable clinical AI models and their translation to hospitals remains difficult. From a computational perspective, these tools must deliver consistent performance across diverse populations and adapt to diverse clinical needs, while learning from biased and scarce data. Moreover, the development of tools relies on our capacity to balance clinical AI utility and patient privacy concerns. In this thesis, I will discuss our contributions in addressing the above challenges in three areas: 1) cancer risk assessment from imaging, 2) personalized screening policy design and 3) private data sharing through neural obfuscation. I have demonstrated that our clinical models offer significant improvements over the current standard of care across globally diverse patient populations. The models now underlie prospective clinical trails.
Date issued
2022-05Department
Massachusetts Institute of Technology. Department of Electrical Engineering and Computer SciencePublisher
Massachusetts Institute of Technology