Leveraging Structure and Knowledge in Clinical and Biomedical Representation Learning
Author(s)
McDermott, Matthew B. A.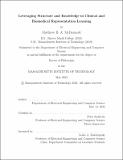
DownloadThesis PDF (6.514Mb)
Advisor
Szolovits, Peter
Terms of use
Metadata
Show full item recordAbstract
Datasets in the machine learning for health and biomedicine domain are often noisy, irregularly sampled, only sparsely labeled, and small relative to the dimensionality of the both the data and the tasks. These problems motivate the use of representation learning in this domain, which encompasses a variety of techniques designed to produce representations of a dataset that are amenable to downstream modelling tasks. Representation learning in this domain can also take advantage of the significant external knowledge in the biomedical domain. In this thesis, I will explore novel pre-training and representation learning strategies for biomedical data which leverage external structure or knowledge to inform learning at both local and global scales. These techniques will be explored in 4 chapters: (1) leveraging unlabeled data to infer distributional constraints in a semi-supervised learning setting; (2) using graph convolutional neural networks over gene-gene co-regulatory networks to improve modelling of gene expression data; (3) adapting pre-training techniques from natural language processing to electronic health record data, and showing that novel methods are needed for electronic health record timeseries data; and (4) asserting global structure in pre-training applications through structure-inducing pre-training.
Date issued
2022-05Department
Massachusetts Institute of Technology. Department of Electrical Engineering and Computer SciencePublisher
Massachusetts Institute of Technology