A Subject Based Methodology for Measuring Interclass Bias in Facial Recognition Verification Systems’
Author(s)
Peña-Alcántara, Aramael Andrés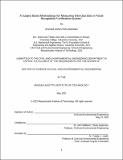
DownloadThesis PDF (7.852Mb)
Advisor
Williams, John
Terms of use
Metadata
Show full item recordAbstract
Rapid progress in automated facial recognition has led to a proliferation of the use of algorithms to support decision-making in high-stakes applications, such as immigration and border control, hiring, and the criminal justice system. Recent research has uncovered serious concerns about equality and transparency in facial recognition algorithms, finding performance disparities between groups of people based on their phenotypes, such as gender presentation and skin tone. These challenges can result in loss of employment opportunities, extra scrutiny in transactions, and even loss of freedom, raising the need of deeper analysis of facial recognition’s shortcomings.
This dissertation proposes a novel methodology and a general test statistic to measure facial recognition algorithm interclass bias. The test uses distance-based variance to capture shape-related differences in an algorithm’s accuracy at multiple operating points.
The author assesses the performance of the test to evaluate the interclass bias for skin tone and gender, in commercial facial verification algorithms. Using a dermatologist-approved classification for skin tone system and a simple masculine and feminine classification for gender presentation, thirteen commercial-off-the-shelf facial verification algorithms are evaluated, utilizing a subset of the IARPA Janus Benchmark C dataset, and it’s 1:1 verification protocol. The analyses show that darker-skinned people have the least accurate results, with interclass bias measures up to 7.2 times higher than lighter-skinned people. Additionally, the results show that one evaluated commercial facial verification algorithm statistically eliminates the interclass bias for skin tone. Yet, all thirteen commercial facial verification algorithms evaluated experienced worse performance for feminine presenting persons compared to masculine presenting persons. The author believes this new measure of interclass bias can be incorporated into an algorithm’s design to remove this bias. The present biases in classifying darker-skinned and feminine presenting people require urgent attention, if commercial companies are to build genuinely equal, transparent, and accountable facial verification algorithms.
Date issued
2022-05Department
Massachusetts Institute of Technology. Department of Civil and Environmental EngineeringPublisher
Massachusetts Institute of Technology