Deep Imbalanced Regression: Challenges, Methods, and Applications
Author(s)
Zha, Kaiwen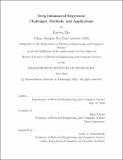
DownloadThesis PDF (1.294Mb)
Advisor
Katabi, Dina
Terms of use
Metadata
Show full item recordAbstract
Real-world data often exhibit imbalanced distributions, where certain target values have significantly fewer observations. Existing techniques for dealing with imbalanced data focus on targets with categorical indices, i.e., different classes. However, many tasks involve continuous targets, where hard boundaries between classes do not exist. We define Deep Imbalanced Regression (DIR) as learning from such imbalanced data with continuous targets, dealing with potential missing data for certain target values, and generalizing to the entire target range. Motivated by the intrinsic difference between categorical and continuous label space, we propose distribution smoothing for both labels and features, which explicitly acknowledges the effects of nearby targets, and calibrates both label and learned feature distributions. We curate and benchmark large-scale DIR datasets from common real-world tasks in computer vision, natural language processing, and healthcare domains. Extensive experiments verify the superior performance of our strategies. Our work fills the gap in benchmarks and techniques for practical imbalanced regression problems.
Date issued
2022-05Department
Massachusetts Institute of Technology. Department of Electrical Engineering and Computer SciencePublisher
Massachusetts Institute of Technology