Decision Making for Populations
Author(s)
Chopra, Ayush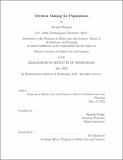
DownloadThesis PDF (22.98Mb)
Advisor
Raskar, Ramesh
Terms of use
Metadata
Show full item recordAbstract
Critical decisions for large populations have conventionally been made top-down, with million-dollar centralized satellites and surveillance tools used to sense events and generate instructions that are enforced on people. The last two years of the pandemic have shown that these methods are ineffective and invade user privacy. With smartphone devices becoming increasingly powerful and users privacy-aware, we need to rethink the future of decision making for populations - to be bottom-up. The overarching theme for this thesis is to build tools that can enable decision making in this new decentralized world of data-driven but privacy-sensitive citizens. We imagine a future where we can preempt and efficiently tackle crises by crowdsourcing sensing significant events using real-time smartphones and engaging people to make population decisions - turning the world into a living lab! To realize this, our technical contributions aim to realistically simulate large heterogeneous populations by privately learning from decentralized data sources. First, we introduce DeepABM a framework for Scalable, Fast and Differentiable Agent-based Modeling. DeepABM can simulate million-size populations in a few seconds on personal computers (up to 300x faster than similar prior-art), enable end-to-end gradient-based optimization and learn from heterogeneous data sources. We validate the design through our epidemiological simulator (DeepABM-Epi), which is used to make policy recommendations for resource-constrained vaccine prioritization and enable in-silico forecasting of infection dynamics by merging simulators with deep neural networks. Second, we introduce Adaptive Split Learning (AdaSplit) a mechanism for distributed & privacy-aware machine learning in low-resource setups. AdaSplit achieves state-of-the-art performance on several distributed machine learning benchmarks and can jointly learn from devices with variable resource budgets. Finally, we evaluate our research through peer-reviewed publications and interdisciplinary collaborations that take a step toward extending this work into impact in the real world.
Date issued
2022-05Department
Program in Media Arts and Sciences (Massachusetts Institute of Technology)Publisher
Massachusetts Institute of Technology